Diopsis AI
Insect recognition
Measuring insect abundance and species diversity has traditionally been done using methods like Malaise traps, light traps, pan traps, pitfall traps, beat sheets, and standardized visual inspections. These methods provide good measurements of insect populations. Still, they are usually lethal for the collected specimens and time-consuming in execution, manual species identification and counting, and therefore often executed at a limited number of sites and periods.
Since 2019, Naturalis is using an improved system: Diopsis V2. This system utilizes a digital camera with a yellow screen to attract insects, capturing images when motion is detected. Powered by solar energy and connected via a 4G network, the DIOPSIS V2 operates autonomously in the field for extended periods. Specialized deep learning software is used for the detection and classification of insects on these images.
Naturalis challenged us to improve these deep learning algorithms, to make identifying insects more efficient. We are developing three different algorithms to do this. The project is not finished yet, but the first results look promising.
If successful, the improved models will be integrated into the DIOPSIS imaging pipeline, further enhancing Naturalis’ capabilities in biodiversity monitoring and research.
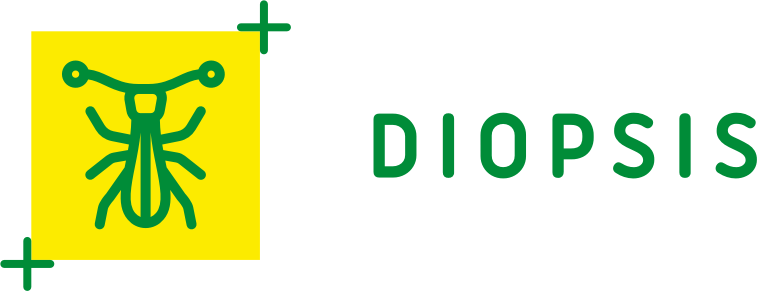
Dive deeper
The challenge is split into two parts: creating a detection model and creating a classification model. The full dataset consists of about 4,000 images of screens, that can be used for detection, and about 50,000 cropped images of insects. All insects are labeled by their taxonomy. This means we have labels of the species of each insect image on different levels of precision.
Classification: We are exploring two classification algorithms: fine-tuning a ResNet model and fine-tuning a CLIP-based model.
Detection: For the detection challenge, we are exploring using YOLOv9. This a model known for it’s outstanding detection capabilities.